Common Actions on Messages in a Queue
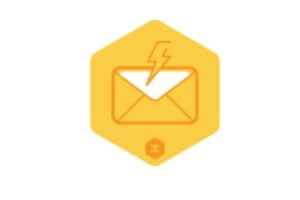
Table of Contents
ToggleOverview
In a previous post, we talked about our ten favorite uses for a message queue. The uses laid out the architectural benefits of putting a message queue in place as a key component within a distributed system.
The writers at HighScalability did us one better with their description of a message queue in their repost of the article. Here’s how they described it:
"[Y]ou can find a message queue in nearly every major architecture profile on HighScalability. Historically they may have been introduced after a first generation architecture needed to scale up from their two tier system into something a little more capable (asynchronicity, work dispatch, load buffering, database offloading, etc). If there's anything like a standard structural component, like an arch or beam in architecture for software, it's the message queue."
We’ve targeted this post at cloud developers who may not have used message queues very much but who are looking for ways to create more robust applications or connect systems together. Other developers may find it useful, though, as a quick refresher. Think of it as Message Queues 201.
Table of Contents:
Achieve Cloud Elasticity with Iron
Speak to us to find how you can achieve cloud elasticity with a serverless messaging queue and background task solution with free handheld support.
Processing
Processing Requests In the Background
One of the more fundamental actions of a message queue in a modern cloud app is triggering backend processing tasks. For tasks that either don't need to happen within the user response loop or that can take place concurrently with additional user actions, it makes a lot of sense to send it to the background and process it asynchronously. Examples might be uploading documents, converting photos, processing credit cards, or collecting analytics.
Rather than address actions serially as part of the user response loop, each action can be sent to some sort of worker that takes care of the processing. Common worker frameworks include Celery, Resque, and our own IronWorker. Message queues serve as the core of these worker systems by buffering the task load and providing a mechanism for distributing the work across multiple cores and servers.
Processing Big Data
Big data processing is far more than just Hadoop. The map-reduce pattern fits only a portion of large-scale processing needs, and Hadoop can sometimes be more complicated and with a steeper learning curve than is absolutely necessary for the task at hand.
Developers need easy ways to run their code in a highly parallel ways. Message queues in combination with scalable worker systems provide a rich platform to do massive processing without having to master new languages or complicated frameworks. A master or control task can split up data segments into manageable slices and queue up the hundreds or thousands of tasks that need to operate on those slices. These workers can put results in cache or database storage. Additional workers can be queued to consolidate results or do other actions on the derivative data.
Every application with large numbers of users or that is doing any processing in the background needs this type of large-scale parallel processing solution. Hadoop isn’t going away, but using robust message queues and high-throughput task queues to do large-scale parallel processing will address the vast number of big data problems that don’t fit a Hadoop model.
Delaying Processing
Often actions need to be delayed to allow other, parallel tasks to finish their work first. Message queues (and worker systems) are often a good way to do this. Most queue systems provide a feature to put a message on the queue with a delay. The delays are, more often than not, short-term delays where it makes sense as part of the processing flow to create a distinct action. Any long term delays should probably be handled outside of a queue structure using scheduled jobs.
Buffering
Buffering for Databases
Many times you need to persist data, but it doesn’t necessarily need to be (or shouldn’t be) persisted as part of the request loop. Etsy’s Statsd is a good example of this scenario: it’s important that stats get persisted, but there are big advantages to bundling a lot of these stats before persisting them. By using a queue, you can ensure that data will get persisted, while still getting the benefits of bundling the data. This lowers the number of database requests, open file handlers, and database load required to persist your data.
Collecting User and Log Events
Collecting Webhook Events
Collecting Data from Connected Devices
Iron.io Serverless Tools
Speak to us to learn how IronWorker and IronMQ are essential products for your application to become cloud elastic.
Thanks for subscribing! Please check your email for further instructions.

Orchestrating Process Flows
Orchestrating process flows is not just about decoupling processes but about providing a framework to chain work together, handle exceptions, and escalate issues. Task-level processing has become virtualized, which means tasks can execute across any number of servers and even in multiple zones or clouds. Message queues are the key way to not just buffer and scale the background processing, but also coordinate the different tasks and the exceptions that might take place.
Chaining Work Together
Handling Exceptions
Escalating Issues
Integrating Independent Systems
Transforming Data
Notifying Services
Buffering Data Updates
Routing Actions
Message Queues as Core Elements

Unlock the Cloud with Iron.io
Find out how IronWorker and IronMQ can help your application obtain the cloud with fanatical customer support, reliable performance, and competitive pricing.

About Iron
1 Comments
Leave a Comment Cancel Reply
This site uses Akismet to reduce spam. Learn how your comment data is processed.
Interestingly enough, I am currently implementing most of these actions inside a .Net desktop app.